기계 학습을 이용한 구인두암의 수술 후 치료 예후 예측
Machine Learning Algorithms for Predicting Treatment Outcomes of Oropharyngeal Cancer After Surgery
Article information
Trans Abstract
Background and Objectives
This study analyzed data from patients who were diagnosed with human papilloma virus (HPV)-associated oropharyngeal (OPC) and treated surgically to construct a machine learning survival prediction model.
Subjects and Method
We retrospectively analyzed the clinico-pathological data of 203 patients with HPV-associated oropharyngeal squamous cell carcinoma (OPSCC) from 2007 to 2015.
Results
In the Cox proportional hazard (CPH) model, the c-index values for the training set and the test set were 0.81 and 0.59, respectively. The univariate analysis showed that contralateral lymph nodes (LNs) metastasis, lymphovascular invasion, pN, stage, surgical margin status, histologic grade, pT, and the number of metastatic LNs had significant correlations with survival. Contrastively, the multivariate analysis showed pT and histologic grade to have significant correlation with survival. In the random survival forest model, the c-index values for the training set and the test set were 0.83 and 0.87, respectively. In the DeepSurv model, the cindex values for the training set and the test set were 0.75 and 0.83. Among the three models mentioned above, Random Survival Forest and DeepSurv showed the best performance for predicting the survival of HPV-associated OPSCC patients.
Conclusion
We confirmed that a survival prediction model using machine learning and deep learning algorithms showed reasonable survival estimates for HPV-associated OPSCC patients.
Introduction
Human papilloma virus (HPV)-associated oropharyngeal squamous cell carcinoma (OPSCC) shows distinctive features compared to head and neck squamous cell carcinoma (HNSCC), typically caused by smoking and drinking [1-3]. Unlike traditional HNSCC, HPV-associated OPSCC occurs in the younger patients, and has a favorable response to treatment and a good prognosis, resulting in a long life expectancy after the termination of treatment. Therefore, the quality of life after treatment should be considered when selecting treatment modalities for HPV-associated OPSCC patients; indeed, clinical studies on de-escalation therapy are currently in progress to reduce the morbidity associated with treatment for this reason [4-8]. In general, many of HPV-associated OPSCC patients have a good prognosis, but 1%-15% develop locoregional failure and 10%-20% die from distant metastasis. In the revised 8th TNM staging system, a distinct staging system was proposed for HPV-associated OPSCC due to its status as an independent disease entity [9,10]. The 8th TNM staging system has a higher correlation with the overall survival of HPV-associated OPSCC patients compared to the 7th staging system, but some patients still show treatment outcomes that are not consistent with their TNM stage [9,11,12]. Therefore, it is necessary to construct a more robust predictive model that can more accurately predict treatment outcomes of these patients.
When surgical treatment is performed on HPV-associated OPSCC patients, the pathological characteristics of each tumor can be analyzed through pathological examination of surgical specimens. Tumor aggressiveness can be estimated through pathological information, such as surgical margin status, number of metastatic lymph nodes (LNs), extranodal extension (ENE), perineural invasion (PNI), lymphovascular invasion (LVI), and lymph node ratio (LNR). In order to create a robust model for predicting OPSCC patient survival, clinical information, including these pathological data, must be integrated in the model. As machine learning and deep learning technologies develop, they are being used in various ways in the medical field. Especially with the development of image recognition technology, corresponding studies have been carried out in the fields of dermatology, radiology, and ophthalmology [13-18]. However, studies to construct machine learning models to predict the treatment outcomes of HPV-associated OPSCC patients are rare. If we establish a robust model for predicting the survival of patients, high-risk patients can be selected, treated intensified according to their risk group, and actively surveilled. This study analyzed the data of patients diagnosed with HPV-associated OPSCC who got surgical treatment at our hospital, calculated the prognostic significance of pathological factors, and predicted treatment outcomes using a machine learning or deep learning model based on clinico-pathologic factors, including those parameters mentioned above.
Subjects and Methods
This study was approved by the Institutional Review Board (IRB) in Yonsei University (2020-1123-002) and was conducted in accordance with the Declaration of Helsinki. Since the IRB waived the need for individual informed consent, it was not obtained from the participants. Data from patients diagnosed with oropharyngeal cancer and treated at Severance Hospital from January 2007 to December 2015 were retrospectively analyzed. Since 2007, we have performed p16 immunohistochemistry (IHC) tests on all oropharyngeal cancer patients to investigate their HPV status. This study included only cases of patients with oropharyngeal cancer who had a positive p16 IHC test and underwent surgery as initial treatment. Exclusion criteria were as follows: 1) prior surgery or radiotherapy on head and neck; 2) distant metastasis at the time of diagnosis; 3) cases lost during follow-up after surgery. Finally, 203 patients, 173 males and 30 females, were included in the study. The patients’ ages ranged from 30 to 81 years, with a mean age of 57.3 years. Staging of cancer was based on the 8th American Joint Committee on Cancer.
When analyzing the prognosis of cancer patients, certain metrics, such as accuracy and area under receiver operating characteristic curve, do not reflect the characteristics of the survival data. The survival data of cancer patients do not consist of binary data, such as survival or death, but rather include elements called censored data and “time to event.” Harrell’s c-index is used as a method for predicting survival, reflecting how well the model predicts patient survival time. c=0.5 indicates the average value of the random model, and c=1 means perfect time-to-death prediction [19,20].
Supervised machine learning was performed using a Cox proportional hazard (CPH) model, random survival forest, and deep learning based-model (DeepSurv). We ran training on the above three models and compared the performance of each of the three models using c-index. Eighty percent of the entire data set was separated as a training set for learning, and the other 20% was used for validation. Compared to the CPH model, which uses a linear combination of variables, random survival forest is a non-parametric survival analysis, and all collected variables are used for analysis to automatically evaluate nonlinear effects between variables and reduce variances and biases [21,22]. The version 0.14.0 of scikit-survival was used to construct random survival forest model. The DeepSurv is an Python module that updates weights using a multilayer feed forward network and back propagation process to presents the negative log partial likelihood as output values [20].
Patient information, pathologic findings, tumor location and stage, recurrence, death, cause of death, and date of death were analyzed using R program version 4.03. An two-sample t-test was used to assess differences in continuous variables between two independent groups. Fisher’s exact test or chi-square test were used to assess differences in categorical variables between two groups. A Kaplan-Meier method was used to analyze survival of patients, while outcomes were evaluated with a log-rank test.
Results
Clinical information
A total of 203 patients underwent neck dissection along with surgical excision of the primary lesion. 112 patients (55.2%) had a history of smoking. Pathological examination showed positive margins in 68 patients (33.5%) and negative margins in 135 patients (66.5%). LVI was observed in 70 patients (34.5%), PNI was observed in 22 patients (10.8%), and ENE was observed in 94 patients (46.3%). For pT classification, 57 patients (28.1%) were classified as T1, 107 (52.7%) as T2, 26 (12.8%) as T3, and 13 (6.4%) as T4. In pN classification, 34 patients (16.7%) were classified as N0, 123 (60.6%) as N1, and 46 (22.7%) as N2. In the 8th TNM staging system, 130 patients (64.0%) were classified as stage I, 61 patients (30.0%) as stage II, and 12 patients (6.0%) as stage III. When we analyzed the overall survival of patients according to tumor stage, there were significant differences between stage III and other stages, while no significant difference was observed between stage I and II (Fig. 1A) (stage I vs. stage II, p=0.56; stage I vs. stage III, p<0.005; stage II vs. stage III, p<0.005). The summary of clinico-pathological characteristics of patients and the baseline differences between training and test datasets were indicated in Table 1. The differences in survival rates between the two groups were analyzed by the Kaplan-Meier method with a log-rank test (Fig. 1B). There was no significant difference between training and test dataset.
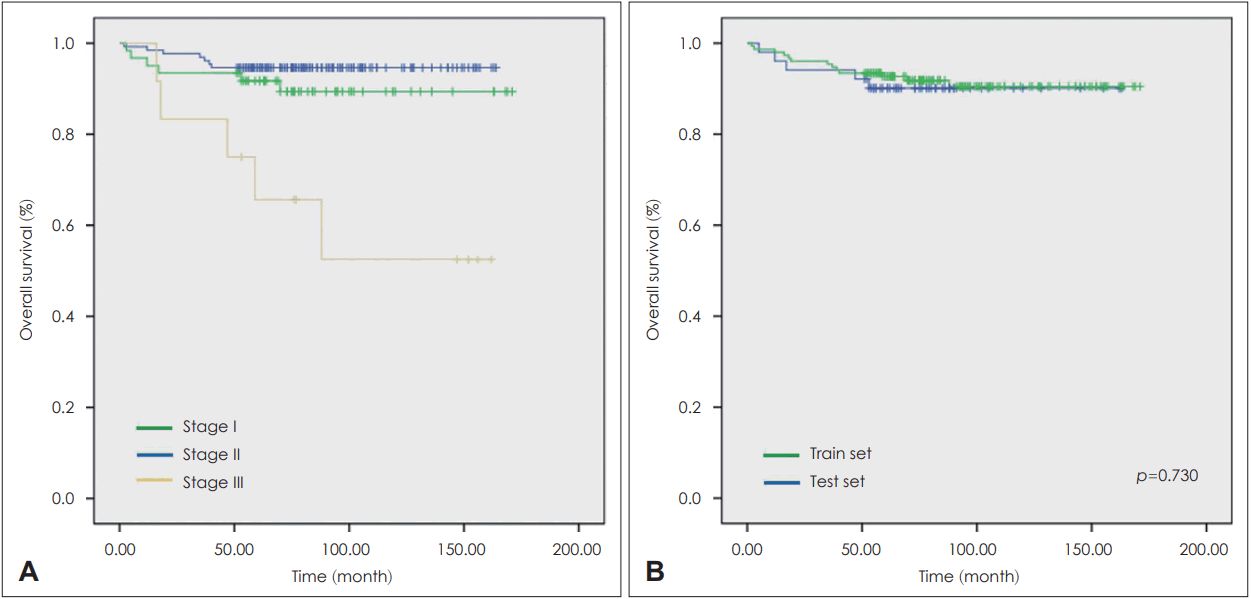
Kaplan-Meier curve. A: The results using the 8th TNM stage of all oropharyngeal squamous cell carcinoma patients. B: The results of training and test sets.
CPH model
Parameters for build a prediction model included the patient’s age, sex, smoking history, primary tumor site, margin status, pT, pN, LVI, PNI, ENE, ipsilateral LNs metastasis, contralateral LNs metastasis, LNR, and adjuvant treatment. When the predictive model was constructed in the CPH model using all the parameters mentioned above, the training set had a c-index value of 0.81 and the test set had a c-index value of 0.59. On univariate analysis, contralateral LNs metastasis, LVI, pN, stage, surgical margin status, histologic grade, pT, and number of metastatic LNs had significant correlation with survival. On multivariate analysis, pT and histologic grade showed a statistically significant correlation with patient survival (Fig. 2). As we used GridSearchCV to tune parameter values, optimal performance was achieved when only margin status, pT, pN, ipsilateral LNs metastasis, and LNR were included in the CPH model. When the CPH model was constructed with these five parameters, the training set had a c-index value of 0.70 and the test set had a c-index value of 0.80.
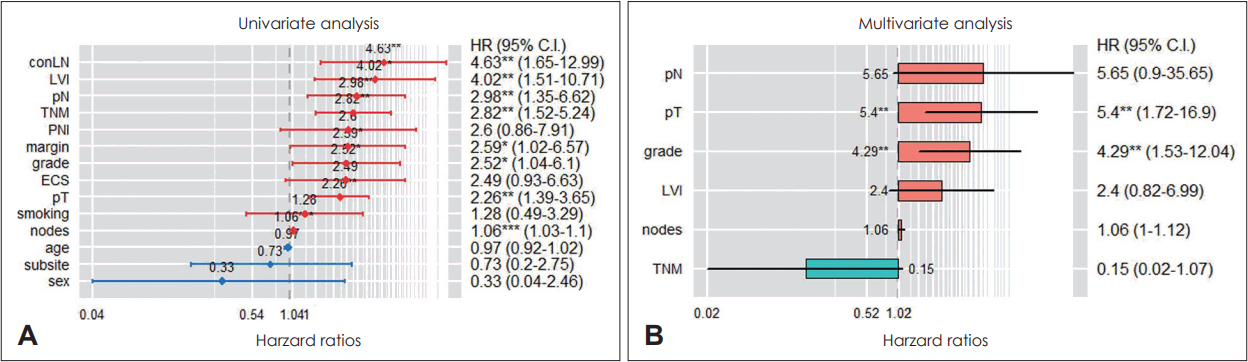
Cox proportional hazard model. A: The results of univariate analysis. B: The results of multivariate analysis. *p<0.05; **p<0.01; ***p<0.001 TNM, stage; grade, histologic grade; margin, surgical margin status; nodes, number of metastatic LNs; ECS, extracapsular spread; conLN, contralateral LNs metastasis.
Random survival forest model
In random survival forest model, the training set had a c-index value of 0.83 and the test set had a c-index value of 0.87. Through an analysis of permutation variable importance (VIMP), the importance of various parameters used in this model construction was analyzed (Fig. 3). The parameters which showed high feature importance in random survival forest was as follows, in descending order: age, grade, number of metastatic LNs, pT, TNM stage, contralateral LNs metastasis, and adjuvant treatment. pT and histologic grade showed significant importance in both CPH and random survival forest models. No variable showed associations with other variables when interactions between variables were measured based on minimum depth.
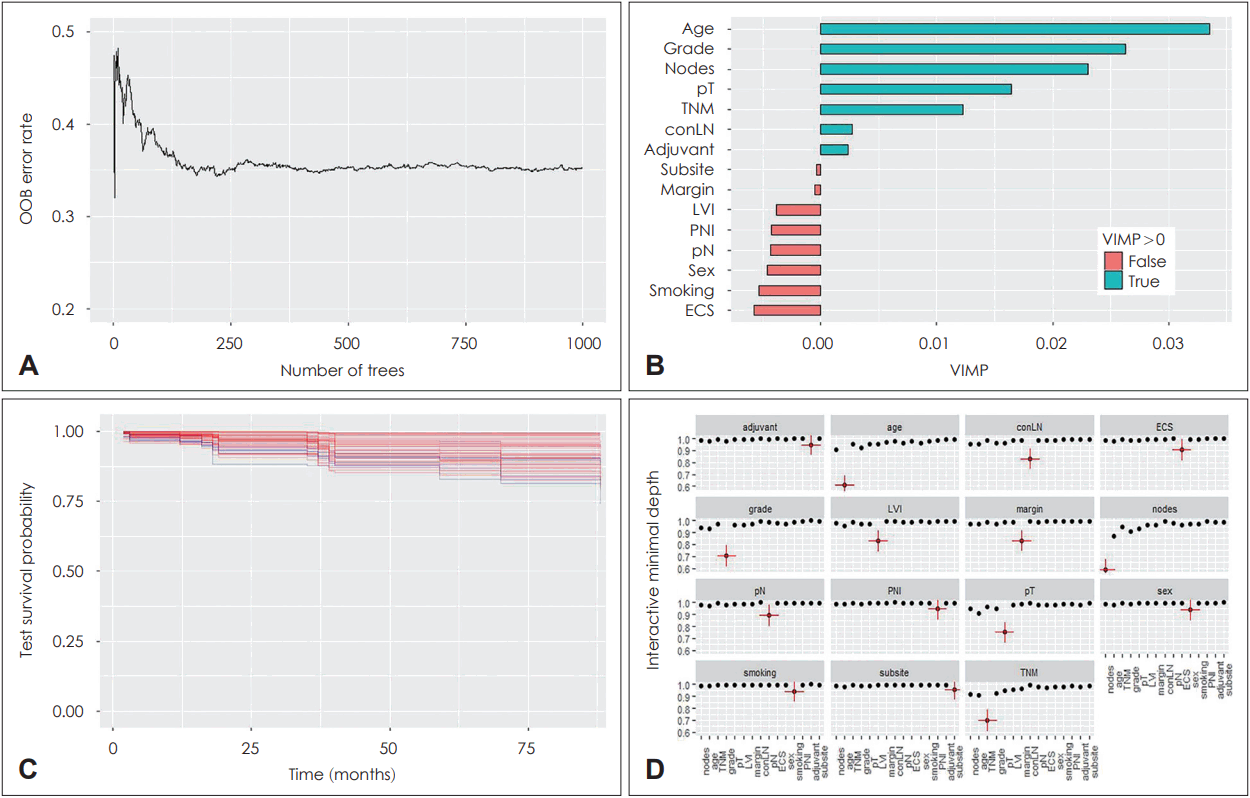
Random forest survival model. A: Random forest OOB prediction error estimates as a function of the number of trees in the forest. B: Estimated survival of testing set. C: Variable importance (VIMP). Blue bars indicate positive VIMP, red indicates negative VIMP. Importance is relative to positive length of bars. D: Variable interaction plot. TNM, stage; grade, histologic grade; margin, surgical margin status; nodes, number of metastatic LNs; ECS, extracapsular spread; conLN, contralateral LNs metastasis.
Deep learning-based model
In the case of the DeepSurv model, the training set had a c-index value of 0.75 and the test set had a c-index value of 0.83. The process of the deep learning is shown in Fig. 4. Both Random Survival Forest and DeepSurv models showed high performance for predicting the survival of HPV-associated OPSCC patients.
Discussion
Accurate prognostication of HNSCC patients is essential for appropriate counseling and personalized precision treatment. To date, prognostication of HNSCC has been performed using a traditional TNM staging system reflecting the characteristics of tumor-node-metastasis. However, as new biomarkers and prognostic factors for HNSCC have been discovered, development of a prognostic system with improved performance is required. In particular, the incidence of HPV-associated OPSCC has been rapidly increasing in recent years. Moreover, given that HPV-associated OPSCC has a distinct clinical course and prognosis, a new staging system according to HPV positivity was proposed in the 8th TNM staging system. In addition, several prognostic systems for OPSCC associated with HPV have been developed and reported [23-25]. In the Denmark system, the patient’s age, performance status, smoking status, HPV status, treatment method, etc. were used as input factors, while age, sex, stage, treatment method, etc. were used in the Erasmus system to predict OPSCC patients’ survival. Each research team proposed a model to predict the prognosis of HPV-associated OPSCC patients based on their input factors, respectively [24]. When compared to the previous TNM staging systems, these models showed higher results with regards to the c-index value, which is the most used index for performance evaluation of survival prediction models. However, in some cases, the results obtained using these models do not agree with each other, so there remain certain limitations to improving the accuracy of predicting the survival of OPSCC patients.
In this study, machine learning and deep learning algorhithms were used to construct a prediction model for survival of HPV-associated OPSCC patients. The c-index values of Random Survival Forest model and deep learning-based models were 0.87 and 0.83, respectively. We demonstrate that, even when compared to the Erasmus or Denmark systems, which showed higher c-index values than the TNM staging system, our machine learning and deep learning-based models showed high performance in terms of survival prediction performance of HPV-associated OPSCC patients. Currently, many studies are ongoing for deintensified therapy in HPV-associated OPSCC patients to reduce the morbidity related to treatment in low risk patients, and it is expected that more accurate personalized treatment will be possible if low-risk groups can be classified using developed machine learning or deep learning prognostic models. Of course, the performance of machine learning and deep learning models should be improved by conducting further research on a larger number of patients, and the effectiveness of each model should be verified through multicenter clinical trial. Nonetheless, in this report we elucidate the possibility of using machine learning models to generate prediction models for survival of OPSCC patients.
Since this study was conducted on HPV-associated OPSCC patients who recieved surgery, various data obtained through pathological examination of the surgical specimen after surgery were used. Factors including margin status, LVI, PNI, number of metastatic LNs, LNR, and ENE were used as input for constructing machine learning model. Also, various clinical factors such as the patient’s age, sex, and adjuvant treatment, were also used as input factors. Given that, unlike traditional statistical methods, machine learning and deep learning models can construct non-linear models of variables, they are less affected by multicolinearity between variables, so a robust prediction model can be created by using a variety of variables as input factors while reducing bias and variance. In the future, if parameters that can reflect the biological behavior of tumors can be extracted from imaging tests, such as CT, MRI, and PET, and incorporated into our model, the performance of our models will be much more improved for clinical application.
This study has the following limitations. As the data of patients who received surgical treatment in a single institution were analyzed retrospectively, selection bias may not have been avoided. Also, since our study used the relatively small number of patients, which was insufficient to elucidate a robust performance of our machine learning and deep learning models, further studies are needed to improve the performance of our models using large-scale data sets. Additionally, since our study only examined patients who underwent surgery as their initial treatment, it may be necessary to verify whether the same performance can be achieved in patients who underwent non-surgical treatment. Lastly, although various clinical pathological factors were used as input factors for our machine learning and deep learning models, follow-up studies are needed to discover and verify new biomarkers that are related to the prognosis of HPV-associated OPSCC.
In conclusion, we confirmed that a survival prediction model using machine learning and deep learning algorithms showed reasonable estimates of survival for HPV-associated OPSCC patients. Although multicenter and large-scale studies should be conducted to make more robust predictive model, we expect that risk stratification using a machine learning model will enable personalized treatment.
Acknowledgements
This study was supported by a new faculty research grant from Yonsei University College of Medicine (2020-32-0047).
Notes
Author Contribution
Supervision: Se-Heon Kim, Eun Chang Choi, Jae-Yol Lim, Yoon Woo Koh. Writing—original draft: Dachan Kim. Writing—review & editing: Young Min Park.